KT AIVLE/Daily Review
241030
bestone888
2024. 10. 30. 19:46
241030
딥러닝
In [1]:
import pandas as pd
import numpy as np
import matplotlib.pyplot as plt
import seaborn as sns
from sklearn.model_selection import train_test_split
from sklearn.metrics import *
from sklearn.preprocessing import StandardScaler, MinMaxScaler
from keras.models import Sequential
from keras.layers import Dense, Input
from keras.backend import clear_session
from keras.optimizers import Adam
In [2]:
path = 'https://raw.githubusercontent.com/DA4BAM/dataset/master/advertising.csv'
adv = pd.read_csv(path)
adv.head()
Out[2]:
TVRadioNewspaperSales01234
230.1 | 37.8 | 69.2 | 22.1 |
44.5 | 39.3 | 45.1 | 10.4 |
17.2 | 45.9 | 69.3 | 9.3 |
151.5 | 41.3 | 58.5 | 18.5 |
180.8 | 10.8 | 58.4 | 12.9 |
In [3]:
target = 'Sales'
x = adv.drop(columns = target)
y = adv.loc[:, target]
In [4]:
# 데이터 분할
x_train, x_val, y_train, y_val = train_test_split(x, y, test_size=0.2, random_state = 1)
# scaling
scaler = MinMaxScaler()
x_train = scaler.fit_transform(x_train)
x_val = scaler.transform(x_val)
In [5]:
# feature 개수
nfeatures = x_train.shape[1]
# 메모리 정리
clear_session()
# Sequential 모델
model = Sequential([Input(shape = (nfeatures,)),
Dense(1)])
# 모델 확인
model.summary()
In [6]:
# compile
model.compile(optimizer = 'adam', loss = 'mse')
# 학습
model.fit(x_train, y_train)
# 예측
y_pred = model.predict(x_val)
# 검증
print(mean_absolute_error(y_val, y_pred))
print(mean_absolute_percentage_error(y_val, y_pred))
print(root_mean_squared_error(y_val, y_pred))
5/5 ━━━━━━━━━━━━━━━━━━━━ 1s 7ms/step - loss: 210.2498
2/2 ━━━━━━━━━━━━━━━━━━━━ 0s 109ms/step
15.052200520802291
1.0141108422779446
15.64066154625038
In [10]:
# compile
model.compile(optimizer = Adam(learning_rate = 0.001), loss = 'mse') # learning_rate default 값은 0.001
# 학습
model.fit(x_train, y_train, epochs = 50, validation_split= 0.2, verbose = 0) # epochs default 값은 1, validation_split은 0.0
# 예측
y_pred = model.predict(x_val)
# 검증
print(mean_absolute_error(y_val, y_pred))
print(mean_absolute_percentage_error(y_val, y_pred))
print(root_mean_squared_error(y_val, y_pred))
2/2 ━━━━━━━━━━━━━━━━━━━━ 0s 22ms/step
14.1109373408556
0.9482398194975982
14.696283366817203
그래프 그리기
In [11]:
path = 'https://raw.githubusercontent.com/DA4BAM/dataset/master/Carseats.csv'
data = pd.read_csv(path)
data.head()
Out[11]:
SalesCompPriceIncomeAdvertisingPopulationPriceShelveLocAgeEducationUrbanUS01234
9.50 | 138 | 73 | 11 | 276 | 120 | Bad | 42 | 17 | Yes | Yes |
11.22 | 111 | 48 | 16 | 260 | 83 | Good | 65 | 10 | Yes | Yes |
10.06 | 113 | 35 | 10 | 269 | 80 | Medium | 59 | 12 | Yes | Yes |
7.40 | 117 | 100 | 4 | 466 | 97 | Medium | 55 | 14 | Yes | Yes |
4.15 | 141 | 64 | 3 | 340 | 128 | Bad | 38 | 13 | Yes | No |
In [12]:
# 데이터 분리
target = 'Sales'
x = data.drop(target, axis=1)
y = data.loc[:, target]
# 가변수화
cols = ['ShelveLoc', 'Education', 'US', 'Urban']
x = pd.get_dummies(x, columns = cols, drop_first = True)
# 데이터 분할
x_train, x_val, y_train, y_val = train_test_split(x, y, test_size= 0.2, random_state = 1)
# scaling
scaler = MinMaxScaler()
x_train = scaler.fit_transform(x_train)
x_val = scaler.transform(x_val)
In [22]:
# feature 개수
nfeatures = x_train.shape[1]
# 메모리 정리
clear_session()
# Sequential 모델
model2 = Sequential([Input(shape = (nfeatures,)),
Dense(1)])
# 모델 확인
model2.summary()
In [23]:
# compile
model2.compile(optimizer = Adam(learning_rate = 0.1), loss = 'mse')
# 학습
history = model2.fit(x_train, y_train, epochs = 30, validation_split = 0.2, verbose = 0).history
# 예측
y_pred2 = model2.predict(x_val)
# 검증
print(mean_absolute_error(y_val, y_pred2))
print(mean_absolute_percentage_error(y_val, y_pred2))
print(root_mean_squared_error(y_val, y_pred2))
3/3 ━━━━━━━━━━━━━━━━━━━━ 0s 15ms/step
1.7647960083484648
284087497942631.1
2.153965030346456
In [24]:
plt.figure(figsize = (10,5))
plt.plot(history['loss'], label = 'train_err', marker = '.')
plt.plot(history['val_loss'], label = 'val_err', marker = '.')
plt.legend(loc = 'upper right')
plt.grid()
plt.show()
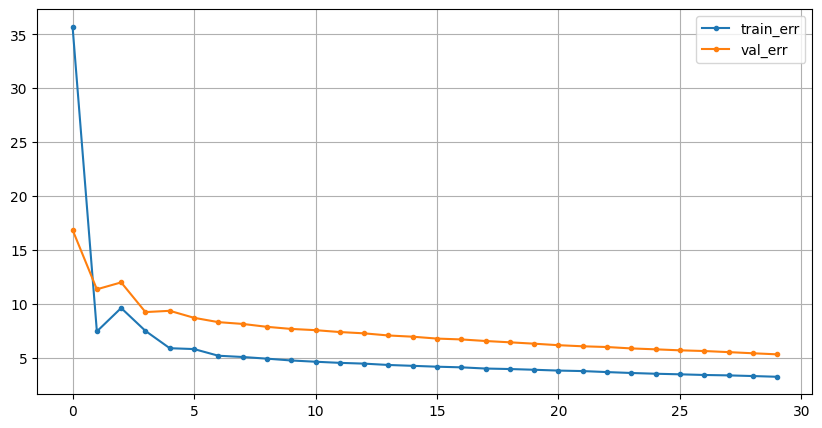
Hidden layer
In [25]:
path = 'https://raw.githubusercontent.com/DA4BAM/dataset/master/boston.csv'
data = pd.read_csv(path)
target = 'medv'
x = data.drop(target, axis = 1)
y = data.loc[:, target]
x_train, x_val, y_train, y_val = train_test_split(x, y, test_size=.2, random_state = 1)
# scaling
scaler = MinMaxScaler()
x_train = scaler.fit_transform(x_train)
x_val = scaler.transform(x_val)
In [29]:
# feature 개수
nfeatures = x_train.shape[1]
# 메모리 정리
clear_session()
# Sequential 모델
model3 = Sequential([Input(shape = (nfeatures,)),
Dense(4, activation = 'relu'),
Dense(1)])
# 모델 확인
model3.summary()
In [30]:
# compile
model3.compile(optimizer = Adam(learning_rate = 0.1), loss = 'mse')
# 학습
history = model3.fit(x_train, y_train, epochs = 100, validation_split = 0.2, verbose = 0).history
# 예측
y_pred3 = model3.predict(x_val)
# 검증
print(mean_absolute_error(y_val, y_pred3))
print(mean_absolute_percentage_error(y_val, y_pred3))
print(root_mean_squared_error(y_val, y_pred3))
4/4 ━━━━━━━━━━━━━━━━━━━━ 0s 10ms/step
3.4524976487253234
0.19954878042560453
4.164142401809952
In [31]:
plt.figure(figsize = (10,5))
plt.plot(history['loss'], label = 'train_err', marker = '.')
plt.plot(history['val_loss'], label = 'val_err', marker = '.')
plt.legend(loc = 'upper right')
plt.grid()
plt.show()
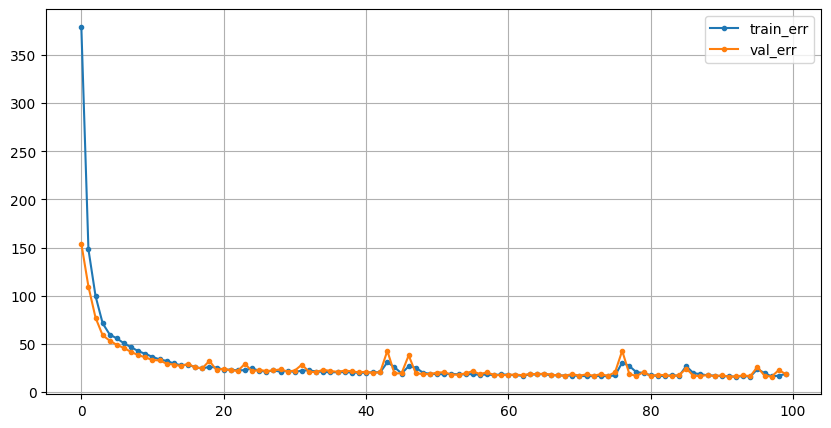
In [31]: