241031
1. 회귀
In [1]:
import pandas as pd
import numpy as np
import matplotlib.pyplot as plt
import seaborn as sns
from sklearn.model_selection import train_test_split
from sklearn.metrics import *
from sklearn.preprocessing import MinMaxScaler
from keras.models import Sequential
from keras.layers import Dense, Input
from keras.backend import clear_session
from keras.optimizers import Adam
In [2]:
path = 'https://raw.githubusercontent.com/DA4BAM/dataset/master/Carseats.csv'
data = pd.read_csv(path)
data
Out[2]:
SalesCompPriceIncomeAdvertisingPopulationPriceShelveLocAgeEducationUrbanUS01234...395396397398399
9.50 | 138 | 73 | 11 | 276 | 120 | Bad | 42 | 17 | Yes | Yes |
11.22 | 111 | 48 | 16 | 260 | 83 | Good | 65 | 10 | Yes | Yes |
10.06 | 113 | 35 | 10 | 269 | 80 | Medium | 59 | 12 | Yes | Yes |
7.40 | 117 | 100 | 4 | 466 | 97 | Medium | 55 | 14 | Yes | Yes |
4.15 | 141 | 64 | 3 | 340 | 128 | Bad | 38 | 13 | Yes | No |
... | ... | ... | ... | ... | ... | ... | ... | ... | ... | ... |
12.57 | 138 | 108 | 17 | 203 | 128 | Good | 33 | 14 | Yes | Yes |
6.14 | 139 | 23 | 3 | 37 | 120 | Medium | 55 | 11 | No | Yes |
7.41 | 162 | 26 | 12 | 368 | 159 | Medium | 40 | 18 | Yes | Yes |
5.94 | 100 | 79 | 7 | 284 | 95 | Bad | 50 | 12 | Yes | Yes |
9.71 | 134 | 37 | 0 | 27 | 120 | Good | 49 | 16 | Yes | Yes |
400 rows × 11 columns
In [3]:
# 가변수화
x = data.drop(columns = 'Sales')
y = data['Sales']
x = pd.get_dummies(x, columns = ['ShelveLoc', 'Education', 'US', 'Urban'], drop_first = True)
In [4]:
# 데이터 분할
x_train, x_val, y_train, y_val = train_test_split(x, y, random_state = 1, test_size = 0.3)
# 스케일링
scaler = MinMaxScaler()
x_train = scaler.fit_transform(x_train)
x_val = scaler.transform(x_val)
In [5]:
# 모델링
nfeatures = x_train.shape[1]
clear_session()
model1 = Sequential([Input(shape = (nfeatures, )),
Dense(2, activation = 'relu'),
Dense(1)])
model1.summary()
In [6]:
# 컴파일, 학습
model1.compile(optimizer = Adam(learning_rate = 0.01), loss = 'mse')
hist1 = model1.fit(x_train, y_train, epochs = 500, validation_split = 0.3, verbose = 0).history
In [7]:
plt.plot(hist1['loss'], label = 'train_err', marker = '.')
plt.plot(hist1['val_loss'], label= 'val_err', marker = '.')
plt.grid()
plt.legend()
plt.show()
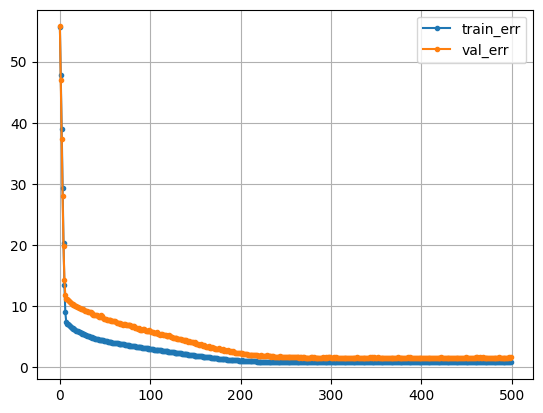
In [8]:
pred1 = model1.predict(x_val)
print(mean_absolute_error(y_val, pred1))
4/4 ━━━━━━━━━━━━━━━━━━━━ 0s 11ms/step
0.9141374322573343
2. 이진분류
In [9]:
path = "https://raw.githubusercontent.com/DA4BAM/dataset/master/Attrition_train_validation.CSV"
data = pd.read_csv(path)
# 숫자형 데이터로 변경
data['Attrition'] = np.where(data['Attrition']=='Yes', 1, 0)
data.tail()
Out[9]:
AttritionAgeBusinessTravelDepartmentDistanceFromHomeEducationEducationFieldEmployeeNumberEnvironmentSatisfactionGender...OverTimePercentSalaryHikeRelationshipSatisfactionStockOptionLevelTotalWorkingYearsTrainingTimesLastYearWorkLifeBalanceYearsAtCompanyYearsInCurrentRoleYearsWithCurrManager12451246124712481249
0 | 27 | Travel_Rarely | Research & Development | 19 | 3 | Other | 1619 | 4 | Male | ... | No | 11 | 1 | 2 | 7 | 3 | 3 | 7 | 7 | 7 |
0 | 29 | Travel_Rarely | Research & Development | 9 | 3 | Life Sciences | 1558 | 3 | Male | ... | No | 18 | 1 | 2 | 5 | 2 | 2 | 1 | 0 | 0 |
0 | 29 | Non-Travel | Sales | 2 | 3 | Life Sciences | 469 | 4 | Male | ... | No | 14 | 1 | 1 | 4 | 3 | 2 | 4 | 3 | 2 |
0 | 43 | Travel_Rarely | Sales | 16 | 3 | Marketing | 327 | 4 | Female | ... | Yes | 22 | 3 | 1 | 22 | 3 | 3 | 17 | 13 | 9 |
0 | 26 | Travel_Rarely | Research & Development | 3 | 4 | Medical | 1177 | 1 | Male | ... | No | 22 | 2 | 1 | 8 | 2 | 3 | 8 | 7 | 7 |
5 rows × 26 columns
In [10]:
# 불필요한 변수 제거
data.drop(columns = 'EmployeeNumber', inplace = True)
x = data.drop(columns = 'Attrition')
y = data['Attrition']
# 가변수화
dum_cols = ['BusinessTravel','Department','Education','EducationField','EnvironmentSatisfaction','Gender',
'JobRole', 'JobInvolvement', 'JobSatisfaction', 'MaritalStatus', 'OverTime', 'RelationshipSatisfaction',
'StockOptionLevel','WorkLifeBalance' ]
x = pd.get_dummies(x, columns = dum_cols ,drop_first = True)
In [11]:
# 데이터 분할
x_train, x_val, y_train, y_val = train_test_split(x, y, test_size = 0.3, random_state = 1)
# 스케일링
scaler = MinMaxScaler()
x_train = scaler.fit_transform(x_train)
x_val = scaler.transform(x_val)
In [12]:
nfeatures = x_train.shape[1]
nfeatures
Out[12]:
53
In [13]:
# 모델링
clear_session()
model2 = Sequential([Input(shape = (nfeatures, )),
Dense(8, activation = 'relu'),
Dense(4, activation = 'relu'),
Dense(1, activation = 'sigmoid')])
model2.summary()
In [14]:
# 컴파일, 학습
model2.compile(optimizer = Adam(learning_rate = 0.001), loss = 'binary_crossentropy')
hist2 = model2.fit(x_train, y_train, epochs = 50, validation_split = 0.2, verbose = 0).history
In [15]:
plt.plot(hist2['loss'], label = 'train_err', marker = '.')
plt.plot(hist2['val_loss'], label= 'val_err', marker = '.')
plt.grid()
plt.legend()
plt.show()
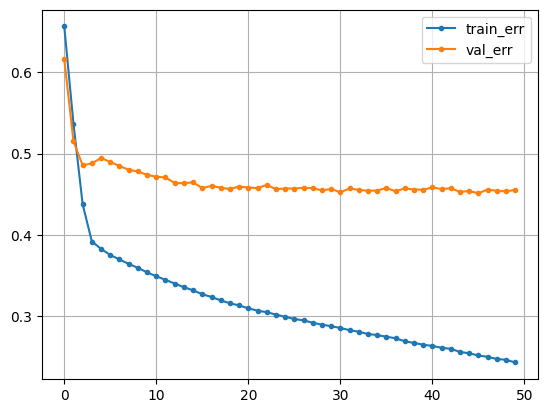
In [16]:
# y값 전처리
pred2 = model2.predict(x_val)
pred2 = np.where(pred2> 0.5, 1, 0)
print(classification_report(y_val, pred2))
12/12 ━━━━━━━━━━━━━━━━━━━━ 0s 4ms/step
precision recall f1-score support
0 0.87 0.98 0.92 315
1 0.67 0.23 0.35 60
accuracy 0.86 375
macro avg 0.77 0.61 0.63 375
weighted avg 0.84 0.86 0.83 375
3. 다중분류
In [17]:
path = "https://raw.githubusercontent.com/DA4BAM/dataset/master/iris.csv"
data = pd.read_csv(path)
data['Species'] = data['Species'].map({'setosa':0, 'versicolor':1, 'virginica':2})
data.head()
Out[17]:
Sepal.LengthSepal.WidthPetal.LengthPetal.WidthSpecies01234
5.1 | 3.5 | 1.4 | 0.2 | 0 |
4.9 | 3.0 | 1.4 | 0.2 | 0 |
4.7 | 3.2 | 1.3 | 0.2 | 0 |
4.6 | 3.1 | 1.5 | 0.2 | 0 |
5.0 | 3.6 | 1.4 | 0.2 | 0 |
In [18]:
# 데이터 분할
x = data.drop(columns = 'Species')
y = data['Species']
x_train, x_val, y_train, y_val = train_test_split(x, y, test_size = 0.3, random_state = 1)
# 스케일
scaler = MinMaxScaler()
x_train = scaler.fit_transform(x_train)
x_val = scaler.transform(x_val)
In [19]:
nfeatures = x_train.shape[1]
nfeatures
Out[19]:
4
In [25]:
# 모델링
clear_session()
model3 = Sequential([Input(shape = (nfeatures, )),
Dense(3, activation = 'softmax')])
model3.summary()
In [26]:
# 컴파일, 학습
model3.compile(optimizer = Adam(learning_rate = 0.1), loss = 'sparse_categorical_crossentropy')
hist3 = model3.fit(x_train, y_train, validation_split = 0.3, epochs = 50, verbose = 0).history
In [27]:
plt.plot(hist3['loss'], label = 'train_err', marker = '.')
plt.plot(hist3['val_loss'], label= 'val_err', marker = '.')
plt.grid()
plt.legend()
plt.show()
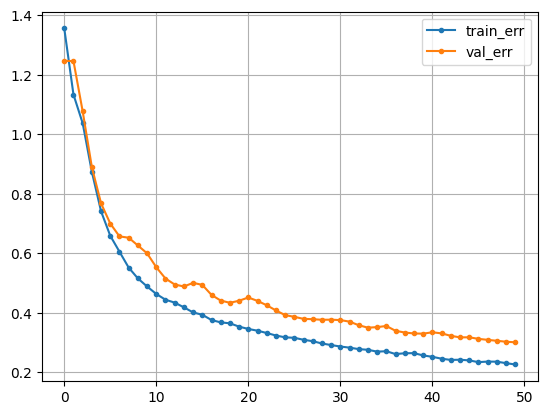
In [30]:
# y값 전처리
pred3 = model3.predict(x_val)
pred3 = pred3.argmax(axis = 1)
print(classification_report(y_val, pred3))
2/2 ━━━━━━━━━━━━━━━━━━━━ 0s 7ms/step
precision recall f1-score support
0 1.00 1.00 1.00 14
1 0.94 0.89 0.91 18
2 0.86 0.92 0.89 13
accuracy 0.93 45
macro avg 0.93 0.94 0.93 45
weighted avg 0.94 0.93 0.93 45
In [ ]:
'KT AIVLE > Daily Review' 카테고리의 다른 글
241104 (0) | 2024.11.04 |
---|---|
241101 (0) | 2024.11.03 |
241030 (0) | 2024.10.30 |
241029 (0) | 2024.10.30 |
241021 ~ 241022 (0) | 2024.10.28 |